How to Implement RAG Effectively in AI Systems
Implementing Retrieval-Augmented Generation (RAG) is more than just adding a new feature to your AI system—it’s a strategic upgrade that enhances the system's ability to generate accurate, reliable, and real-time responses. By adopting best practices, organizations can maximize the benefits of this advanced technology.
Best Practices for RAG Implementation
- Choose Trusted Data Sources: Select reliable databases, APIs, or repositories to ensure that the AI retrieves accurate and relevant information.
- Optimize Retrieval Processes: Balance retrieval and generation to avoid overloading the system. Focus on retrieving the most critical data for efficient and accurate responses.
- Prioritize Data Security: Ensure that all retrieved data is processed securely and complies with privacy regulations, especially in sensitive industries like healthcare and finance.
- Leverage Scalable Infrastructure: KOLO_AI® uses Microsoft Azure to provide secure, scalable, and efficient RAG implementations, ensuring smooth operations even with high query volumes.
Example: RAG in Action
Consider a customer support system:
- Query: "Can you check the status of my order?"
- RAG Response: The AI retrieves the latest order status from the company’s database and provides an instant update to the customer.
Security and Compliance
KOLO_AI® integrates robust security measures to ensure that RAG implementations meet industry standards for data protection and compliance. This guarantees safe and trustworthy operations for businesses.
Recommended Articles
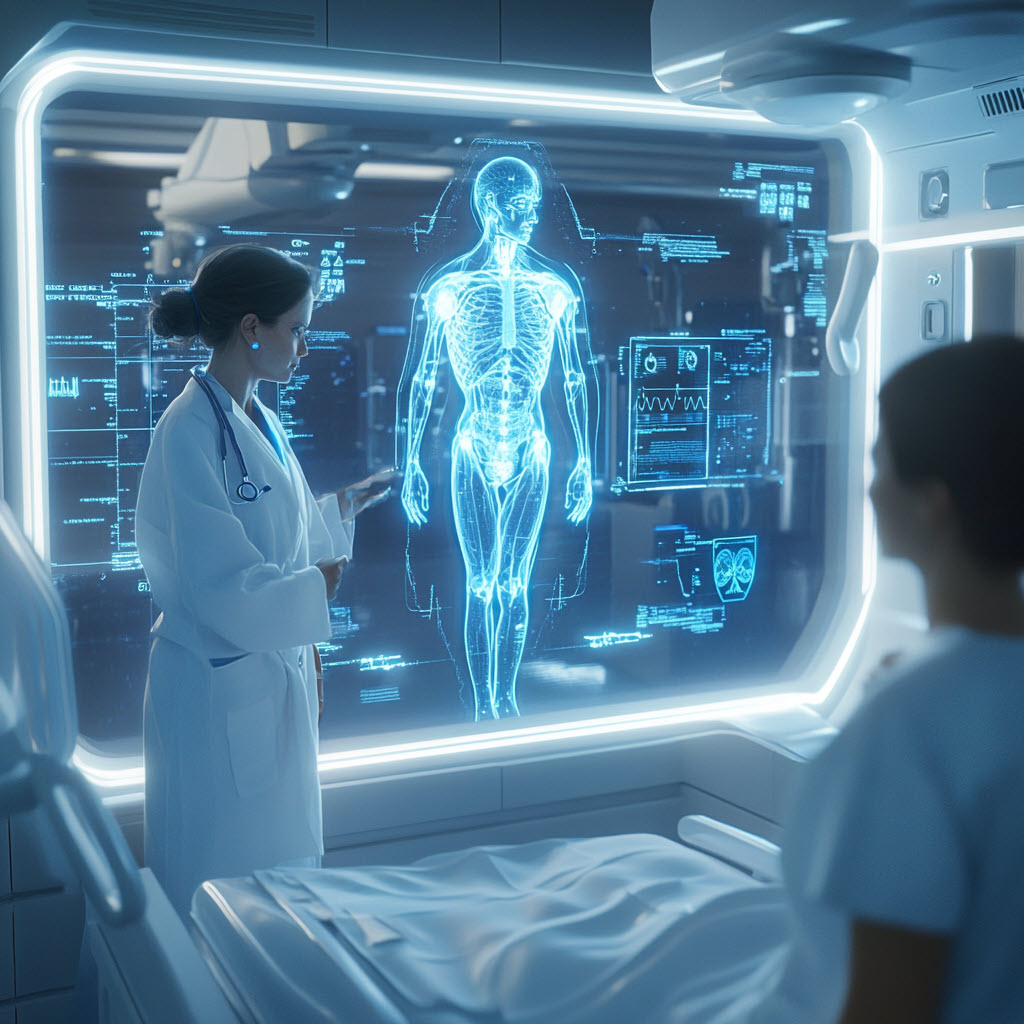
AI in Healthcare: Safeguarding Medical Advice with RAG
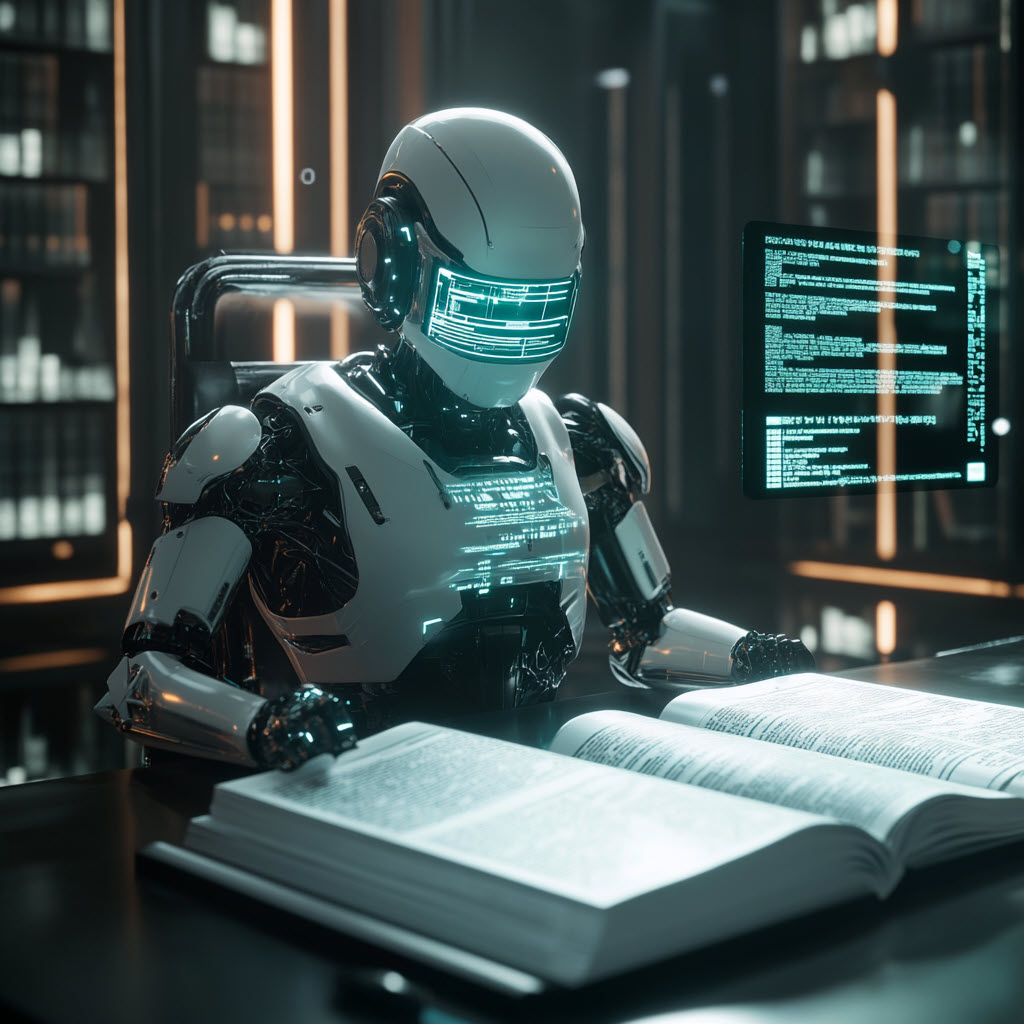
AI for Legal Advice: Why Safe AI-Powered Responses Matter
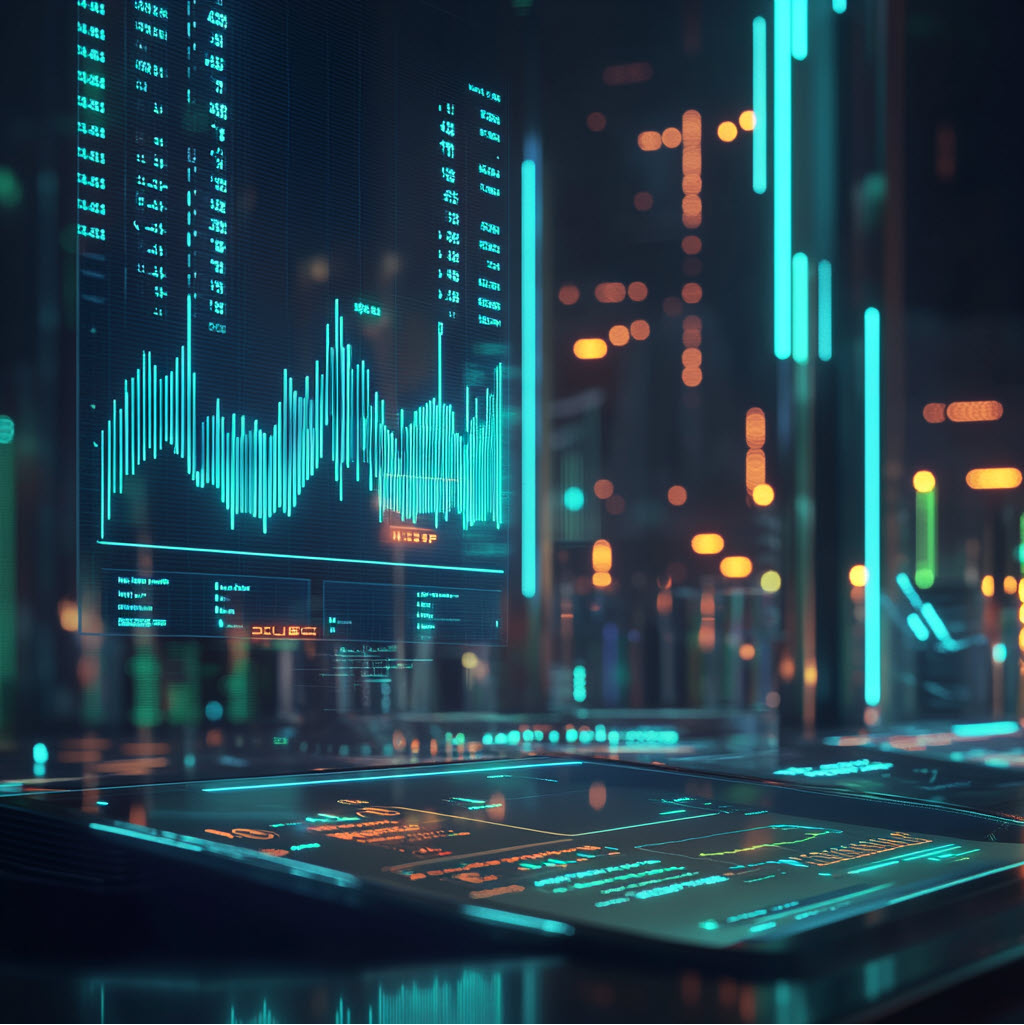